KEY CONCEPTS
•
Predictive maintenance programs may require significant initial and ongoing investments in sensors, software, personnel and training.
•
Setting up a program requires customers and vendors to prioritize key operations and data, understand individual mechanical systems and components and know what conditions should trigger an alert.
•
Automation efforts are evolving, but today’s computerized monitoring and data analysis systems still require humans with knowledge and experience to provide context, interpretation and verification.
From household budgets to multibillion-dollar infrastructure projects, the same question always comes up: “If we spend more now, will it be worth it in the long run?” When the subject is mechanical systems, budget choices often include “run to failure,” maintenance on a fixed schedule or condition-based monitoring and predictive maintenance. The first two options are simple and require little in the way of initial investment, but unexpected failures can be expensive or even dangerous. The third option often involves significant investments in staffing resources, sensors and software (at least, for the time being). For critical assets and large operations, however, the long-term benefits can more than justify the initial costs.
One form of predictive maintenance uses onboard sensors to monitor and report a machine’s condition and software to track trends and anomalies in sensor readouts and provide alerts when something needs attention. A well-planned predictive maintenance program can save money and extend equipment life by reducing unnecessary maintenance and by alerting staff to potential failures between regularly scheduled inspections.
1 Data archives provide a performance history, alerting maintenance crews when it’s time to pre-order replacement parts and lubricants, helping them avoid lengthy interruptions in service. A data archive also provides an auditable source of documentation to back up warranty claims and prove adherence to industry standards.
2
Getting started
Predictive maintenance programs can involve hundreds of sensors producing thousands of data points daily, so a good software analytics system is a necessity. The amount of time and customization required to set up a predictive analytics system varies by vendor, says Daniel Paul, reliability engineer/mechanical supervisor at Catalyst Paper, a large paper and pulp manufacturer in British Columbia, Canada. He notes that trials using predictive analytics for continuous monitoring and condition-based maintenance are a work in progress. “It’s where we’re wanting to move to,” he says, “but it’s easier said than done.”
Setting up a predictive analytics system requires collecting and uploading data to train the system’s software and customizing various set points that trigger the alarms
(see Setting Up Your System).3 Component specifications and operating speeds must be considered, as well as threshold values for triggering an alert. “If you make a template for one system and put it through to all of them, you might not actually be getting good data back,” Paul says. Setting the alarm thresholds too low can result in too-frequent alerts, and setting them too high poses a risk of missing situations that require attention.
Setting up your system
Even though costs of predictive maintenance systems and services are coming down as the technology becomes more readily available, they can be expensive to set up and run. Thus, it makes sense to start a predictive maintenance program with a few durable assets that require the most attention.
3
Have someone in house who understands the system and knows when an automated alert signals a real problem rather than a vehicle running over a rough patch of ground or a processing machine in a warm area of the plant. A real-time alert that isn’t seen until several hours later might or might not be a problem, depending on the type of operation. For situations that are likely to require an immediate response, well-trained staff should be on site during all hours of operation.
Start collecting data on all monitored assets as soon as possible, using a standardized format and procedure. This data serves as the input for the monitoring algorithm, and it provides a baseline that defines normal operating conditions. Knowledge of your operation’s systems and what constitutes abnormal conditions is key to setting alarm thresholds effectively.
As your staff becomes more familiar with the system, get their input on how to improve the predictive maintenance program.
2 Are alarms coming in too often or not often enough? Are there other assets that would benefit from inclusion in the program? Are there other types of data that should be collected or other analyses that should be performed?
One advantage to using sensors and software to monitor a system is that data can be collected more frequently—hourly or even every second, for example—than is typical for a human engineer who might take manual readings, say, once a month. Although the software is programmed to send an email or text message when it registers an abnormal condition, a human engineer must still look at the data and evaluate whether any action is required, Paul says, and this could occur several hours after the software flags a potential problem. In some cases, though, this is an advantage, he says, because the engineer has several readings on which to base a decision rather than just one.
Chase Benning, senior project engineer at Metro Vancouver since January 2023, describes setting up a system at his previous job at Lehigh Hanson (now Heidelberg Materials), with sensors that produced data on the scale of 10,000 data points per second. “I was working on a lot of rotating equipment like cement kilns, ball mills, vertical mills, compressors, pumps, belts and conveyors” for this large-scale supplier of cement, aggregates, ready-mixed concrete and asphalt, he says.
Sensors on the compressors reported multiple bearing temperature readouts, ball mill sensors reported simple one-dimensional average vibration readings and various electrical sensors reported voltages and currents
(see Figure 1). Because the predictive analytics setup was already well established, says Benning, “it was no big problem to put another sensor on any piece of equipment.” However, adding more data required decisions on which equipment would benefit most from additional sensors. He notes, for example, that predictive analytics was not a particular necessity for their small fleet of loaders, forklifts and skid-steers.
Figure 1. Onboard sensors signal unusual vibration patterns that can indicate when a bearing is beginning to fail. Figure courtesy of Jinyi Tai, J., et al. (June 2022), “Mathematical biosciences and engineering: MBE,” 19 (8), pp. 8057-8080, CC BY 4.0.
Sensor data was fed into analytical software with a dashboard interface. “It was built in house, and it was quite sophisticated,” Benning says. Users could set up the dashboard to show trends and other displays for specific equipment. They monitored currently active trends or looked up past trends to identify causes of breakdowns or to get a sense of what was typical for a particular piece of equipment. A corporate IT support group and an onsite process control group helped with setup, implementation and support.
Benning notes that setting up this type of program only makes sense if the value of the assets covered and the risk of downtime or product loss is greater than the investment of time, money, tools and staff for implementing and running the program
(see Alternative Approaches). He adds that large operations are more likely to have in-house support for software development, support and training. Sensor installation, maintenance and alert monitoring also require staff resources. “There’s likely no payback on a maintenance program for one piece of equipment,” he says. However, as predictive analytics software becomes more widely available, he adds, it’s easier now for somewhat smaller operations to set up a basic maintenance program. For example, sensors on a small fleet of trucks could keep track of motor temperatures and send alerts whenever certain thresholds were exceeded.
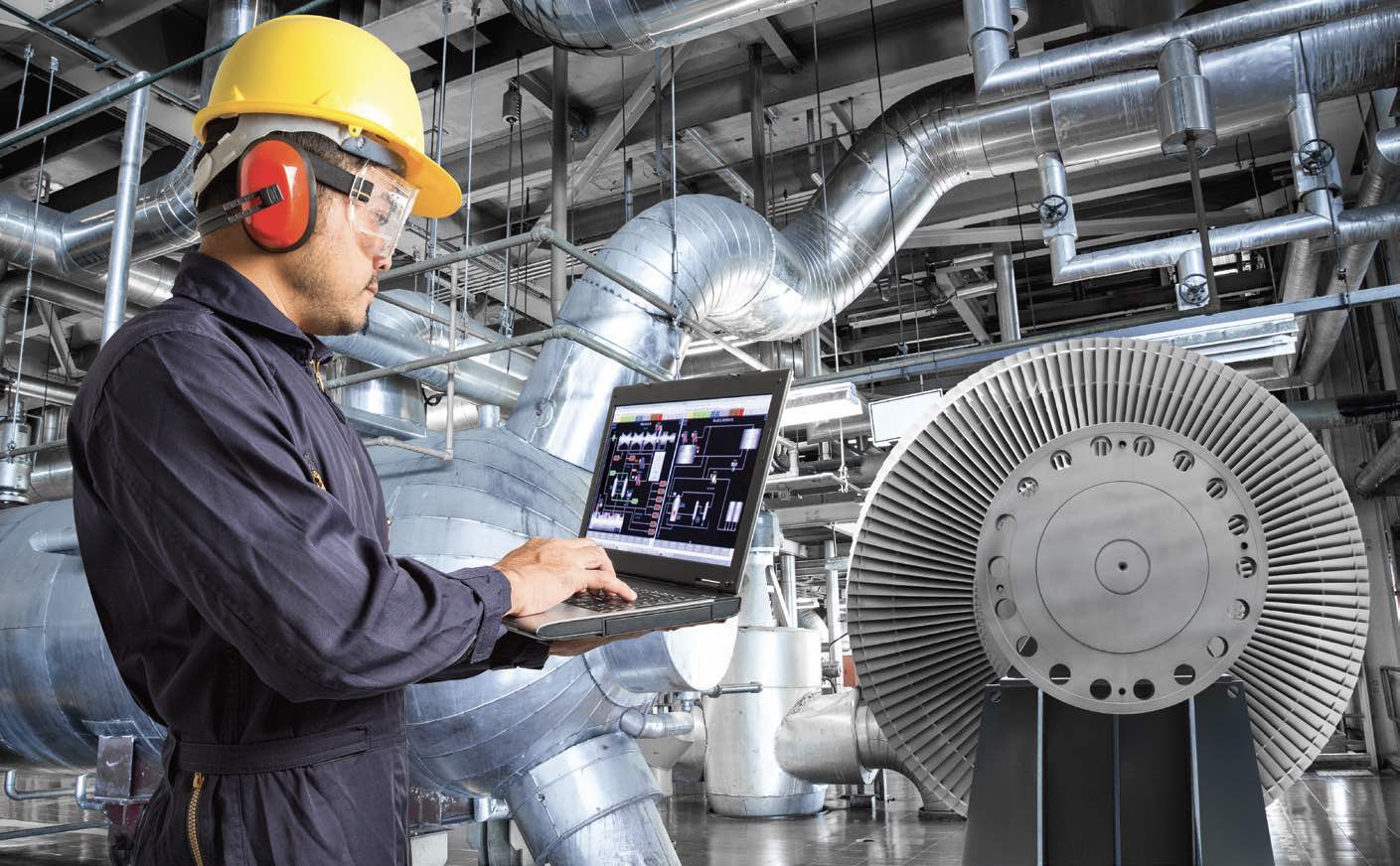
Alternative approaches
Operations with expensive assets, large vehicle fleets or high risks and large costs associated with downtime and product losses stand to gain the most from predictive maintenance. Smaller or less critical operations could benefit more from strategies like condition-based maintenance or advanced troubleshooting. Both of these approaches can be set up to work with onsite sensors that provide real-time data, but they use this data in different ways, and they are less dependent than predictive maintenance on trends and event predictability.
Jory Maccan, reliability fleet analyst for Imperial, and his co-workers are exploring implementation of an asset health index (AHI) program to support their predictive asset maintenance efforts for heavy off-road vehicles.
4 Similar AHI programs are used in the electrical power generation and transmission industry. Rather than scheduling maintenance based on fixed dates, mileage and operating hours, an AHI is based on the criticality of the asset, results of field inspections and laboratory tests, how long an asset or component has been in service and frequency and type of sensor alarms. Tracking an asset’s AHI makes it possible to assign priorities based on risk levels, and thus allocate monetary and workforce resources efficiently. Maccan notes that generally, only high-risk or high-impact assets or components justify the time and effort needed to generate and monitor an AHI.
Although sensors and software can collect and process a mountain of data, collecting all the data all the time is often not the best approach. Selecting data to include in an AHI, Maccan says, requires identifying the types of data that contribute most to making an informed decision. In addition, relevant trends in the data and thresholds for taking action must be identified and quantified4—the length and severity of a structural crack and threshold values that signal a high risk of failure, for example. Relevant operating conditions include extremes of temperature, humidity or dust. Making these decisions requires a thorough understanding of the conditions specific to a given operation’s assets, the performance history of those assets, how asset conditions affect the reliability of the system and how reliability and risk translate into cost impacts.
The sensors: Data in
For an industrial operation where the machines run continuously, automatic sensor readings can offer a real advantage. For example, a machine that is manually checked once a month could develop a problem the day after it’s been checked, and the problem would not be detected until the next reading is taken a month later. “That issue might have been something small that you could have corrected, but by the time you get there, it’s now an issue that you can’t just fix while [the machine is] running. You need to swap parts out,” Paul says. He and his co-workers have seen numerous instances where sensor readings and automatic alerts have helped them quickly locate and confirm the source of a problem, which helped them correct the problem in its early stages. Sensor readouts also indicate when a machine is running normally, saving time on routine manual inspections. “Every day, everyone’s being asked to do more with fewer people,” Paul says, so ensuring efficiency has become a necessity.
Paul adds that, for large operations, the expense of installing and setting up a predictive analytics system can pay for itself quickly. The size of the investment depends on the number of monitoring points being installed, among other things. The payoff depends on the criticality of the system and the potential consequences of downtime due to equipment failure.
1 He adds that, as is the case for other technological developments, these systems are following a trend toward lower costs and greater capabilities. Variables include the costs of buying the sensors or renting them from a supplier, as well as costs involved in licensing fees for the software or in using open-source software and customizing the capabilities in house.
Jory Maccan, reliability fleet analyst for Imperial, works for an oil sands mining operation in Alberta, Canada. He explains that they use lubricating oil condition monitoring based on laboratory analysis of oil samples and inspection of magnetic debris captured by magnetic plugs in the oil stream
(see Figure 2). Periodic engine oil changes for their heavy, off-road vehicles are scheduled based on past experience, he says. “We run fairly consistently with the number of hours that we put on at a time,” he explains, “so we have a really good idea where our engine oil is going to break down.” Previous testing, in which drain intervals were gradually increased, established how long it took for the oil to develop acidity and load with combustion byproducts. This information was used to establish a safe zone for scheduling an oil change, he says, but changing to a different type of oil would require repeating this testing.
Figure 2. Magnetic plugs in the oil stream of heavy, off-road vehicles capture magnetic debris for laboratory analysis. Figure courtesy of Jory Maccan, Imperial.
An analysis program, based on data from previous analyses, allows the lab to rank the conditions of the oil samples using a 1-to-10 scale, Maccan says. These rankings are weighted to account for environmental and operating norms using the history of oil analysis results for similar components under similar operating conditions. Benchmarks and average expected life values for oil, air filters and other components are set based on historical component life records, failure rates and the root causes of failures. This approach requires adherence to standard sampling intervals, data analysis methods and reporting practices. The resulting data can then be used to generate reliable context and interpretations, as well as enabling a comparison of asset conditions across the organization to ensure sound and equitable budget decisions.
4
Onboard sensors provide data to track the performance of the mining equipment, Maccan says. The sensors send alerts to the operation’s dispatch center, and these alerts can be used to create an automated work order, but it takes a human reviewer’s approval before the work order is put through for fulfilment. That way, a failed sensor or a wiring problem does not trigger multiple unnecessary work orders. An on-screen display shows hourly restriction readings that give an early indication of a filter beginning to clog.
Like their off-road counterparts, one of the main uses of onboard sensors in on-road transportation is in reporting the current condition of a vehicle, says Ahmed Mumeni, an expert in public sector fleet electrification in Canada. “Any kind of electric vehicle (EV) is essentially a computer on wheels,” he says. “There’s so much data that’s being generated by these vehicles. If you tap into that data network, you can use that data to conduct any sort of analysis.” For example, the software industry is slowly creating more automated solutions for power management within the transportation sector, he says, because vehicle fleets are incorporating growing numbers of EVs.
Even though fleet managers might have to contract out their data analysis to software companies, they have ample access to raw vehicle data, Mumeni says. He cites SAE J1939 from the Society of Automotive Engineers (SAE International).5 This collection of standards applies to the high-speed controller area networks (CANs) for heavy-duty vehicles, including on-highway transportation. “Something like speedometer data broadcast on a CAN can be generic across vehicles that adhere to SAE J1939 standard,” he explains. A mechanic can plug a data logger into a diagnostic port on the vehicle and download sensor data, or a vehicle with a SIM card can transfer data over an internet connection, often in real time as the vehicle is traveling. Not all types of data are available this way, he adds. For example, manufacturers of EV batteries can encrypt the data that their sensors produce to protect their intellectual property.
Mumeni is contributing to an effort to use energy models to estimate the amount of electricity required to power a fleet of electric buses. This information is used to create master facility designs with charging infrastructure. The energy models take into consideration the battery health of an electric bus, weather and the use of onboard heating and ventilation systems, varying terrain and road conditions, driver behavior, frequency of stops and the number of passengers on board. It’s no trivial task to integrate the many interacting factors that go into sizing and designing the charging infrastructure for a fleet of electric city buses or delivery trucks that could include hundreds of vehicles, he says.
Because buses run on fixed schedules and typically return to their depot at the end of the day, bus depots must have enough charging stations to make sure that all their buses are fully charged and ready to go the next morning. Fortunately, a transit service can save significant amounts of money by charging its vehicles during overnight off-peak hours, when electricity rates are lower. The time it takes for EV batteries to recharge, especially for larger vehicles, is longer than it would take to top off a tank with gasoline or diesel fuel, and this consideration also factors into determining the optimum number of charging stations
(see Figure 3). Vehicle charging data provides valuable information on how long a given size of bus traveling a given type of route can go before it needs another charge, and all these factors must be built into the charging schedule.
Figure 3. An electrically powered city bus recharges at a charging station in New York City. Figure courtesy of Marc A. Hermann (MTA), Wikimedia Commons CC BY 2.0.
Another efficiency measure, Mumeni says, is to warm or cool the bus passenger compartments to the desired temperature while the bus is at the depot, a procedure called preconditioning. Using power from the electrical utility grid to bring the interior temperature of the bus up to the desired setpoint leaves more battery power for propulsion and extends the range that the bus can travel on a single charge. This approach requires the adoption and incorporation of vehicle preconditioning standards built into charge stations at the depot, and sufficient time must be built into the bus schedules to complete the preconditioning.
In addition to energy management, would other sensing and predictive capabilities be useful for large EV fleets? “You basically start with general questions—are the subsystems aboard a given EV functional?” Mumeni asks. “Is the power train system functional? Is the battery pack functional? Is it at the optimal operating temperature? Is there enough coolant onboard that can maintain temperatures of your batteries? Is there enough gear oil in the gearbox?” The maintenance needs of an EV are not as onerous as for an internal combustion engine vehicle, he adds, but outside temperatures affect the range of an EV, and so temperature control of a battery pack is a novel issue for EV fleet operators. Some EVs have transmissions (although they are different from conventional internal combustion engine transmissions), and they require transmission and differential oils.
The software: Data out
Different software systems offer different levels of prediction, Paul says. Systems that take frequent readings can spot trends and patterns in operating conditions. This doesn’t yet reach the level of predicting problems, he says, although algorithms are moving in that direction. In his industry, analytics programs flag bearing defects and wear as they develop, which can indicate a higher risk of failure in the future. He notes that in the automotive industry, where multiple sensors continuously collect and transmit data any time a vehicle is in operation, certain types of vibrations or noises can predict a part failure to a high degree of accuracy. However, he cautions, identifying specific kinds of fault conditions from sensor readouts still requires human interpretation and a knowledge of the system and its operating conditions.
Sensors from different vendors typically generate similar types of readings, Paul says. A vibrational spectrum, for example, will look the same, no matter what vendor supplies the sensor. Where the difference lies is in how a vendor’s software stores and handles this data. A large industrial operation may have one or more systems to collect and analyze sensor data and other systems to archive the data. Still another system may be used to gather and integrate data from various sensors and other sources and generate routine reports and graphs.
For older systems or networks that combine several types of software, transferring data from one part of the system to another in a timely and accurate fashion can be difficult. Many software providers use proprietary codes, making it difficult to port data from one application to another without paying additional licensing fees, Paul says. “It either locks you in with vendor A,” he says, “or it drives you away from them, and you go to someone who has open-source data, because then you can funnel that into another system.”
Maccan works in a newer operation, and all of the computer dispatch technology they use is less than a decade old. This newer commercial software requires fairly standard inputs and outputs, and compatibility across equipment manufacturers is not a particular issue, he says. In addition, their vendors routinely provide software updates and improvements, so they don’t have legacy systems still running, and the sensors they use provide data in a form that is usable by any of the major analytics providers.
Another approach is required when an application is so new that there is a scarcity of data to build on. Large fleets of EVs are still an emerging concept, and real-world data on power management and vehicle performance on this scale is still scarce, says Mumeni. Thus, fleet managers rely on mathematical models to predict energy usage and recharging infrastructure requirements and to optimize their run schedules. Results for one fleet might not be universally applicable because of location-specific factors like seasonal temperature extremes. Mumeni notes that fleet managers often rely on consulting companies that run energy analyses on specific f leets and routes, using their own proprietary models. Extrapolating the results from one analysis to a larger fleet or a different set of routes might not produce accurate results, so the fleet manager must often contract with the consultant for multiple analyses, and the costs can mount up quickly.
The human factor
Implementing the predictive analysis program at his site was easy, Benning says, because there was already an established maintenance program, and the monitoring and analysis software was well built. The staff on site came up to speed quickly after training, and they liked the ease of gathering data and the quality of data presentation. “The decision-making process is still the same; it’s just getting used to the new dashboards,” he adds.
The software can’t do it all, however, Benning says. He cautions: “If you’re looking at the data and not interpreting it, you’re wasting your time.” Some sensor alarms are connected to interlocks, such that when a vibration, temperature or another threshold is exceeded, the equipment automatically shuts down. Other alarms require a human to provide context, based on an understanding of the characteristics of specific pieces of equipment. For example, he says, two presumably identical ball mills operating at the same facility could produce different operating data, so that the analytics required different interpretations.
Benning notes that even automated, software-generated work orders are vetted by maintenance staff before they are carried out. Too many alerts, especially if they are triggered by non-events like routine inspections that involve taking equipment up and down repeatedly, can result in operators ignoring the barrage of emails and texts. Categorizing alarms by urgency can help, he says, but “you can only categorize them so much.”
Machine learning software that learns from the data to make better predictions is beginning to appear, Paul says, but this effort is still in the early stages. A human’s insight is still needed to “close the loop,” he says. That is, when the software indicates that there is a problem, a human confirms whether it actually was a problem and provides information on the severity of the problem. That type of model validation can increase confidence in the model and enable the machine learning algorithm to distinguish between common and uncommon situations.
Automated work orders could work well in applications like an automatic greasing system, Paul says. The system could be set to apply grease at set intervals and monitor a constant stream of ultrasonic signals coming from the sensors. Even here, however, a human is involved in the decision-making process, he says. For example, when a system detects a sensor reading that crosses a preset threshold, it sends a message to an operator to confirm or cancel an instruction to apply more grease to the affected part. The system can be programmed to apply extra grease without getting confirmation first, but it generates a report if a specific part begins to require more frequent grease applications. This allows time for an operator or engineer to inspect the part for a developing problem and to order a spare part and schedule maintenance or repairs if needed. “But the full-on autonomous isn’t quite there yet for us,” he adds.
Automated sensors in inaccessible areas in paper mills can ensure that readings are taken andcrecorded routinely, without the need for an operator to make a special trip to check on machinery that otherwise runs well on its own.
There are locations in a paper mill where no one routinely walks by, Paul says. Automated sensors in these locations can ensure that readings are taken and recorded routinely, without the need for an operator to make a special trip to check on machinery that otherwise runs well on its own. When there’s a potential problem, a human steps in and evaluates the situation, he adds. “How bad is this bearing? You look at the trends,” he says. “Regardless of what the trend shows, I want to go out there and actually touch it and make sure that it’s the item I think it is.” He explains that a paper machine could have 12 bearings within a six-foot radius. An anomalous reading for one bearing might indicate a problem confined to that one bearing, or the reading could be a symptom of a wider problem. “I like to verify before actually getting people to do the work. What needs to be done?”
In an industrial operation that runs 24/7, an inability to port data from one application to another can delay responses to alerts. “If the system tells you that you need to change a bearing, nobody knows except for the one person who got the notification, and you’re waiting for that person to see it,” Paul says. For example, he says, vibration readings from various sensors are sent to the vibration tracking software and to a data archive that all the operators have in their control rooms. An operator may see vibrations increasing for a particular machine, but this information might not be enough to identify a specific problem. Until a qualified person can identify and diagnose the problem, no action can be taken to rectify it. “You need someone to come in and tell you what’s going on,” he says. A system can get bumped, or a bolt fastening an accelerometer to a machine can come loose. A change in temperature or humidity can change a reading. “Some of these machines are in pretty nasty environments,” he says, and so an automated alert might signal something other than an incipient part failure.
Humans also can catch situations that require attention but that don’t set off an automatic alarm. Maccan cites an instance when an anomalous sensor reading signaled a crack in an air intake pipe that was allowing the intake to pull dust into the system. The analyst looking at the screen noticed an outlier in a band of data from multiple sample points and spotted a series of unusually low intake restriction readings. The system, which was set to monitor clogged air filters, did not have an alarm trigger for low restrictions. “Situational awareness is important when you’re looking at that kind of data,” he says.
Unlike the stationary equipment in a factory, mining equipment runs over rough ground, so vibrations are a part of normal operations. “In a mining environment, vibrations have to be significant before they should trigger an alarm,” Maccan says. By that time, damage to a bearing has passed the point of easy interventions, but he notes that they have been able to catch an anomaly in a gear mesh before it failed. “It allowed us to make some adjustments and buy some time.”
Maccan and his colleagues have looked into machine learning applications to enhance their predictive capabilities, but, he says, “we’re just not there yet, where we can trust it and have it work on a scale that we need.” At present, they are using the data they collect to identify failures in the early stages rather than predict them in advance. “It’s still very much eyes on the computer screen and techs on the equipment,” he says. His operation’s maintenance center is staffed 24 hours a day, so if a sensor triggers an alarm, someone is always there to see it and follow up as necessary.
Constant monitoring from a control room is especially necessary, given that much of their equipment runs autonomously. Maccan works in the first operation of its kind in North America to incorporate autonomous equipment on such a large scale. They have learned a lot about best practices from a similar operation in Australia, but they have had to discover other things on their own, he says, like running operations in the Canadian winter weather. “The people we have in dispatch are all heavy equipment mechanics, and they’ve spent time working on the equipment, so they have the maintenance knowledge,” he says.
Display screens in the cabs of loaders, shovels and other equipment that requires a human operator let the operator see immediately if there’s a problem. These alarms can be strictly informational, or they can signal a situation that should be checked into soon. The highest level of severity signals a situation that requires the equipment to be shut down immediately—low engine oil pressure or high coolant temperatures, for example.
Support from system vendors also is a must-have. “We’re looking for vendors and manufacturers with a proven track record,” Maccan says, as well as quality control programs like ISO 9001. “We’re also looking for somebody who is able to step into their process,” he adds, referring to a vendor’s ability to quickly identify a problem with one customer’s system and notify other customers of the problem so that they can take appropriate action before it negatively affects their operations. “If another mine runs into a problem, it’s nice to know that your vendor found the problem and tells you that they isolated the problem.” Often, if a customer reports a problem, the vendor has likely seen it before, and they can make recommendations. “It’s nice to be able to have that kind of supply chain competence,” he says.
Return on investment
For large operations, expensive assets or critical processes, an army of digital assistants to watch over the machinery can be well worth the investment. Continuous data streams—and the software to aggregate, analyze and archive them—can provide valuable insights for maintenance decisions, process optimization and purchasing plans.
Vendors can quickly identify a problem with one customer’s system and notify other customers of the problem so that they can take action before it negatively affects their operations.
Predictive analytics also helps staff communicate better with their management, Benning says. Data and analyses that support the business cases for improvements, accurately tracking downtime and having the analytics on leading indicators of potential failures can help managers to see operational decisions in terms of their business impact.
Computers can’t do it all (yet)—predictive maintenance still requires humans with the knowledge and experience to provide context, interpretation and verification. But as digital systems evolve, their benefits could become accessible to a much greater variety of operations.
REFERENCES
1.
Beckman, M. (2021), Predictive maintenance polishes up mining,” TLT,
77 (1), pp. 38-40. Available
here.
2.
Mudrick, R. (Feb. 21, 2023), “Can predictive maintenance protect your business?” Business News Daily. Available
here.
3.
Aikin, A. R. (2021), “The process of effective predictive maintenance,” TLT,
77 (2), pp. 34-40. Available
here.
4.
Maccan, J., CMRP and Mann, J., M.A.Sc, P. Eng, “The application of the asset health index in oilsands mining,” Canadian Institute of Mining (members-only content).
5.
SAE J1939 Standards Collection. Available
here.
Nancy McGuire is a freelance writer based in Albuquerque, N.M. You can contact her at nmcguire@wordchemist.com.