Turning simulations into formulae by machine learning
By Drs. Wilfred T. Tysoe & Nicholas D. Spencer, Contributing Editors | TLT Cutting Edge February 2023
Machine learning allows the results of complex simulations to be reproduced more rapidly.
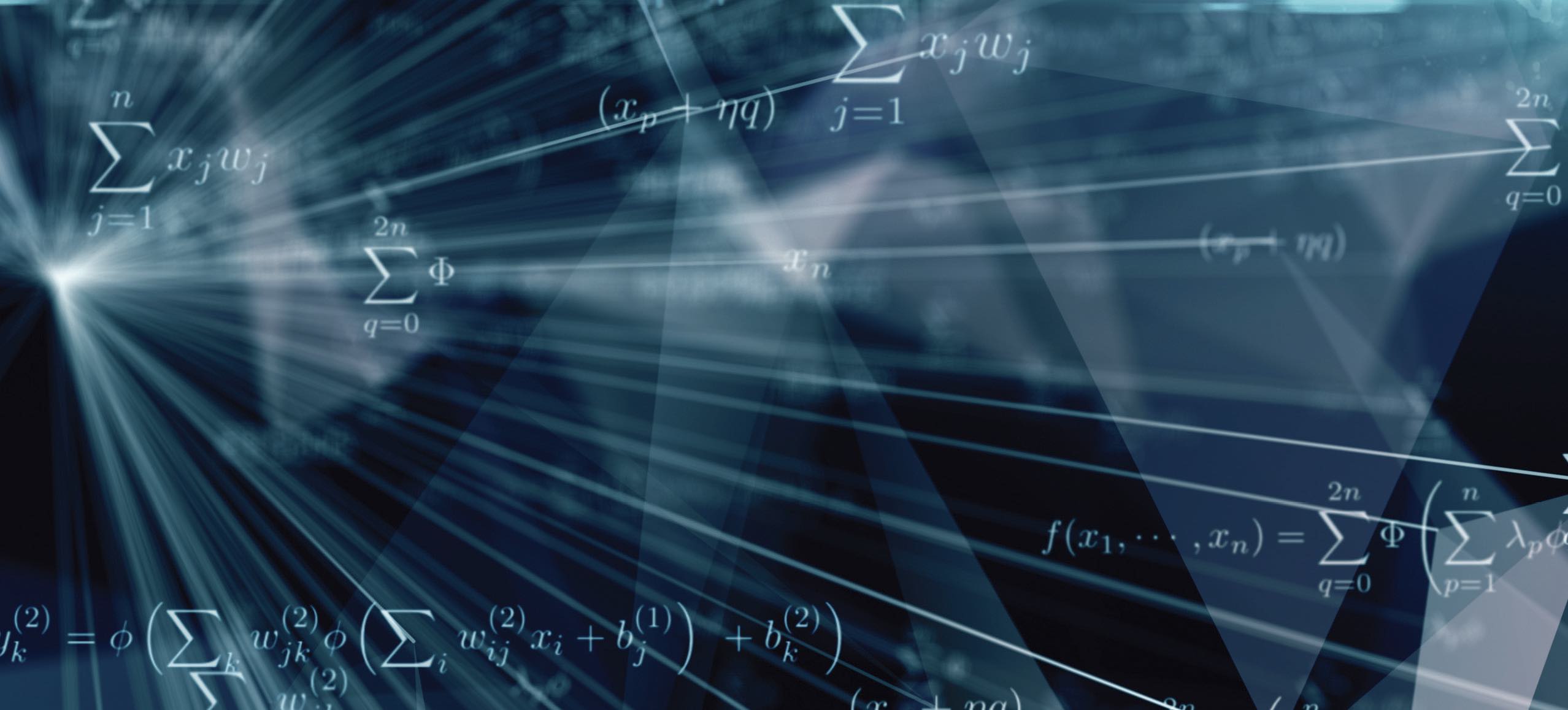
Recent TLT Cutting Edge columns have discussed how machine learning can be used to discover new tribological materials, for example, novel two-dimensional layered materials or friction modifiers.
1, 2 Machine-learning methods work best when there is a large amount of data to fit to the models. This is challenging for tribological measurements because they can be difficult to make and are often made under different conditions, which are often not carefully recorded, or may just be unknown.
However, as recently discussed by Dr. Mahdi Mohammadpour from the Wolfson School of Mechanical, Electrical and Manufacturing Engineering at Loughborough University, working with students Jack Walker, Harry Questa, Anirudh Raman and Muhammad Ahmed in collaboration with Stephen Rickie Bewsher and Günter Offner from AVL List GmbH in Graz, Austria, machine-learning methods may work very nicely to speed up accurate numerical calculations of complex tribological interfaces.
There are generally two approaches to analyzing complex tribological systems. One can either use simplified models that yield approximate answers in a short time or carry out complex numerical calculations that include all interfacial effects in great detail but take a long time and/or significant resources to perform. The group aimed to solve these issues by carrying out precise calculations for a complex sliding interface, in this case for rough, lubricated steel-steel contacts in a roller-element bearing and for a spur gear, to generate a large data set with up to 5,000 data points. Such extensive tribological data sets would be difficult to come by experimentally.
They then used a quite large artificial neural network (ANN) to train a model for the data set using parameters such as the load, entrainment velocity, reduced radius and elastic modulus of the surface, pressure-viscosity coefficient of the fluid, its reference viscosity, the Poisson ratio and density of the substrate, and the contact length. The ANN was originally designed to try to mimic the interconnectivity of neurons in the brain. However, when it is used to model the behavior of complex systems, each node in the network works with the others to try to optimize the parameters efficiently and to improve the agreement between the computer model and the data.
As shown in Figure 1, the agreement between the precise numerical model and the machine-learning results is very good for calculations of the lubricant film thicknesses for the spur gear
(see Figure 1a) and the roller-element bearing
(see Figure 1b). However, the machine-learning model arrived at an answer about 1,500 times faster than the full numerical simulations. Because these results were tested against the data used to train them, the group generated their own independent results for a system that was not used to train the model by measuring the friction coefficient versus entrainment speed for a Group III base oil sliding against AISI 52100 steel. They still found impressive agreement between the model obtained by machine learning, numerical simulations and experiment
(see Figure 1c). Finally, the friction of the spur gear versus angular displacement was calculated, to investigate the sharp decrease in friction expected as the sliding velocity decreases to zero as the gear rotates. As shown in Figure 1d, the machine-learning model reproduces the simulated data very well but ~1,300 times faster than the numerical simulations.
Figure 1. Application of central-film-thickness computation ANN (solid blue) for (a) spur gear, (b) roller-element bearing applications compared against analytical equations (short dash green) and the numerical simulation (long dash red). (c) Comparison between experimentally measured friction coefficient using a mini traction machine (MTM), the computed friction coefficients from the numerical model and the ANN. (d) Application of viscous friction ANN for a spur-gear application compared against analytical equations and the numerical solution. Published with permission from Ref 3.
Perhaps the main use for machine-learning methods in tribology will not be as an approach for designing new materials, but rather as a method for analyzing and optimizing extremely complex tribological interfaces that may improve engineering-design methods. Further advancements in the application of machine learning for tribological analysis will also lead to more detailed predictions of parameters such as pressure, temperature and shear distributions or the subsurface stress field.
REFERENCES
1.
Tysoe, W.T. and Spencer, N.D. (2020), “Designing lubricants by artificial intelligence,” TLT,
76 (6), p. 78. Available
here.
2.
Tysoe, W.T. and Spencer, N.D. (2020), “Designing lubricants by artificial intelligence—the sequel, TLT,
76 (8), pp. 68-70. Available
here.
3.
Walker, J., Questa, H., Raman, A., Ahmed, M., Mohammadpour, M., Bewsher. S.R. and Offner, G. (2023), “Application of tribological artificial neural networks in machine elements,”
Tribology Letters, 71, 3.
Eddy Tysoe is a distinguished professor of physical chemistry at the University of Wisconsin-Milwaukee. You can reach him at wtt@uwm.edu.
Nic Spencer is emeritus professor of surface science and technology at the ETH Zurich, Switzerland, and editor-in-chief of STLE-affiliated Tribology Letters journal. You can reach him at nspencer@ethz.ch.