Meet the Presenter
This article is based on a webinar presented by Auburn University on May 4, 2021. The webinar series, titled The Beard Tribology Webinar Series, is named after the late Ralph Beard, who pioneered the study of tribology at Auburn. Industry 4.0 Tribology-aware, Design for Additive Manufacturing in Energy and Bioengineering Systems is available here.
STLE member C. Fred Higgs, III, is the John & Ann Doerr professor of mechanical engineering and professor of bioengineering at Rice University in Houston, Texas. He is also the faculty director of the Master of Engineering Management and Leadership (MEML) program at Rice University.
Higgs explains, “Tribology enables you to characterize, model and experimentally interpret interfacial sliding behavior in situ.” Rice University has the Additive Manufacturing, Performance, and Tribology (AMPT) Center that sponsors an annual symposium with scholars from around the nation. One of the research labs that is affiliated with the AMPT Center is the Particle Flow & Tribology Laboratory (PFTL), which was founded by Higgs to solve tribology and particle media-related problems.
In the webinar, Higgs explains how the current abundance of digital data available through sensors and the internet is steering problem solving to focus on personalized solutions to engineering problems.
You can reach Higgs at higgs@rice.edu.
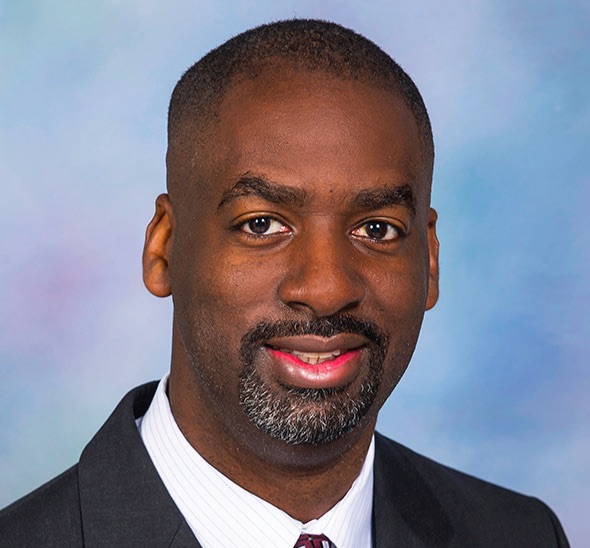
C. Fred Higgs, III
KEY CONCEPTS
•
The current abundance of digital data, which are being generated by sensors, communicated through internet access and translated with artificial intelligence residing in the cloud, is making personalized solutions to engineering problems more commonplace.
•
By using tribology-aware, artificial intelligence-based digital twins of engineering systems, real-world tribological applications can be rapidly designed for additive manufacturing with 3D printing systems.
•
This use of digital data will change education going forward as our world moves from mass production to mass customization through these new technologies and the advanced usage of data.
•
A new generation of Industry 4.0 engineering manager leaders must be produced to fully harness the power of this digital transformation era and the fourth industrial revolution.
Industry 4.0 can use a series of technologies, including autonomous robots, simulation-based engineering, digital twins, the internet of things (IoT), cybersecurity, cloud computing, additive manufacturing (AM), augmented reality and big data. The convergence of these technologies represents the development of a new paradigm.
This article is based on a webinar, titled Industry 4.0 Tribology-aware, Design for Additive Manufacturing in Energy and Bioengineering Systems, and presented by STLE member C. Fred Higgs, III—the John & Ann Doerr professor of mechanical engineering and professor of bioengineering at Rice University in Houston, Texas—and Auburn University. See Meet the Presenter for more information.
According to Higgs, there have been three industrial revolutions, and we are now entering the fourth. Companies thriving in each revolution are a part of that numbered “industry” as follows:
• Industry 1.0: The first revolution involved the development of mechanization and the introduction of steam and water power.
• Industry 2.0: The second revolution began when mass production assembly lines used electrical power to manufacture items like automobiles.
• Industry 3.0: In the third revolution, the assembly lines became automated using computers, information technology (IT) systems and robotics.
• Industry 4.0: Finally, sensors and the internet in combination with the cloud and machine learning have enabled the smart factory and autonomous systems. These systems allow data to interact with machines to direct the machines and improve the process.
In today’s world, there can be an abundance of data available for collection and study. The fullness of the data can allow processes to be completely characterized, and tools like artificial intelligence (AI) enable that “big data” to then be personalized. Higgs believes this new approach will change everything about how people work and live in the world.
Additive manufacturing
Traditional (subtractive) manufacturing begins with solid bulk material. The bulk material is then carved out through subtractive manufacturing to produce a three-dimensional (3D) object and waste material. In contrast, the most industrialized forms of AM begin with powdered material that goes through AM and uses computer-aided design (CAD) to produce a 3D object with only minimal waste material. There is the potential for no geometrical constraints with AM.
Higgs identifies seven main AM or “3D printing” technologies: photopolymerization, material extrusion, material jetting, binder jetting, powder bed fusion (e.g., electron or laser beam fusion), direct energy deposition and sheet lamination. He then expands on fusion and binder jet 3D printing techniques, which are both types of powder bed-based AM technologies.
In one type of powder bed fusion AM technology, the binding mechanism is a high-energy laser source, and this led to AM technologies (e.g., selective laser sintering [SLS], direct metal laser sintering [DMLS] or selective laser melting [SLM]) that selectively fuse the powder particles. Another type of AM technology, binder jetting, uses a fluid binding mechanism where a liquid bonding agent or glue selectively binds regions of a powder bed. The factors impacting powder bed AM technologies include particle effects like particle shape, moisture between particles, aeration and specific energy, confined loading, electromagnetic forces, particle size distribution, particle roughness, particle coverage and particle cohesion.
Binder jet 3D printing involves five steps: powder delivery, powder spreading, binder jetting, sintering/infiltration and build platform lowering to reach the completed build. At the build platform lowering stage, the process can be begun again as needed to reach the completed build stage.
Powder rheometry
Powder rheometry is applied to AM to assess the rheological behavior of the feedstock powders, namely its “spreadability.” A powder rheometer stirs the powder to measure the mount of bulk flow energy needed to push the powder. The energy signature can be correlated to the powder properties, and those properties are needed to calculate the powder properties using the discrete element method (DEM). Higgs explains this research using the example of Ti64 (titanium-64) where powder rheometry experiments showed that increasing particle size and sphericity lead to a decrease in flow energy. This work suggests there might be a threshold for reducing energy and cohesion based on particle size.
The goal of a powder rheometer setup is to progress from real physical experiments to virtual experiments by creating digital twins of both the rheometer and test powder. For example, the Higgs group characterized the physical powder in the rheometer and then built a “digital twin” of the powder by matching the DEM data to the experimental data. Once physical results can be reproduced by the virtual model, a digital twin has been created. This digital twin can then be used in other digital simulations to optimize the spreadability of the powder.
Powder spreading experiments
Most powder bed AM technologies involve a powder spreading step, so elucidating this process is a crucial step in advancing numerous types of 3D printers. While the powder spreading can be optimized through trial and error, optimization can be performed through computer modeling. Modeling can more quickly and cheaply determine the “spreading process recipe” required to uniformly spread powders layer by layer, ultimately aiming for the perfect printing result.
Machine learning can be employed to generate 3D printing recipes by connecting the spreading parameters (e.g., the rotational and/or linear velocity of the spreader) to 3D printing response variables such as surface roughness and powder throughput; this can quickly lead to a process map. Rather than using vast amounts of powder, a test-tube-sized amount of powder is needed to generate the process map that can give the 3D printer settings required to achieve uniform spreading.
Higgs’ lab has developed a proprietary AI-computer modeling tool for developing spreading recipes to produce defect-free 3D printed parts. The spreading recipe can then be tried on the evaluator’s 3D printer. An academic form of the tool also exists as a generic computer model where certain inputs are required, including:
•
the powder to use (e.g., Ti-6Al-4V, stainless steel, cobalt chrome, tungsten carbide),
•
the particle size distribution (e.g., uniform, normal, gamma, log-normal) and
•
the particle size (e.g., 250 μm, 100 μm, 50 μm).
The model will generate a digital twin of the powder. A CAD file is selected to reflect the geometry of the 3D printing spreader as all 3D printers have different geometries (e.g., EOS-like, ExOne-like, Arcam-like, textured roller). Once the recipe is developed, a virtual build is performed to evaluate how the object comes out. If it is distorted, refinements can be made to the process. Once the virtual build matches the desired object, the 3D printing can be started. This effort is intended to optimize the printing process.
One question is on the expense in terms of energy that 3D printing requires. Higgs notes that physical experiment-driven trial and error for 3D printing is expensive in terms of time, money and energy. In contrast, model-guided experimentation allows for the solution to be reached more quickly and efficiently. Creating digital twins of physical phenomena can streamline the printing solution process.
Binder jet printing
In binder jet 3D printing, binder (glue) droplets come down onto the spread powder layer to “bind” the powder, layer by layer, into the desired 3D printed object. Higgs created an experiment to use computational modeling to optimize binder jet printing performance.
Using computer models developed in his laboratory, Higgs and his students studied the binder jet process with different powders at varied droplet frequency.
3 For example, they found that different particle sizes showed less particle movement during the printing process and studied he forces acting on the particles. By incorporating fully coupled fluid-particle interaction physics in the computer model, accuracy can be improved.
In the laboratory, Higgs’ students, in collaboration with students from the lab of Zack Cordero at Massachusetts Institute of Technology (MIT), looked at sintering where the binder itself can crystallize into nanoparticles between the spread powder with the goal of preventing distortion of the post-sintered 3D object.
In situ images revealed up to a 75% reduction in distortion by using these nanoparticles.
1, 2
Applying AM to geothermal energy problems
Higgs provides the example of applying AM modeling to drilling for the clean geothermal energy industry. Higgs’ lab aimed to optimize the drilling process by creating virtual avatars or digital twins of both the rock being drilled and the drill bit. The computer modeling allows the study of both drilling dry and drilling with fluid to evaluate fluid pressure under the drill bit. These digital twins allow for refinement of the drill bit design to optimize the drilling process for each individual drilling situation. The result of the computer modeling study is a 3D-printed drill bit, which has been optimized and tailored to drill a specific rock formation.
A bench-top drilling simulator was used in the laboratory to study friction and wear on the drill bit and the drill’s rate of penetration (RoP) of the rock. Physical drilling experiments on Tennessee sandstone were compared to the modeling results. The results led to a drill bit design chart, which is a process map for 3D printing the drill bit.
These experiments lead to the development of a proprietary AI-guided design software for rapid-excavation and wear-resistant drill bits. An academic form of the software exists as a computer modeling framework. Along with Rice research scientist and STLE member Prathamesh Desai, who also earned his doctoral degree in Higgs’ lab, the design for additive manufacturing (DFAM) framework has led to software that incorporates AI into the production of custom tools and devices.
The model allows the user to select the type of rock (e.g., Crab Orchard sandstone, Castlegate sandstone, granite, shale), the grain size distribution (e.g., uniform, normal, gamma, log-normal) and the particle size (e.g., 500, 250, 100 μm). The model then performs some characterization tests leading to virtual drilling to generate a rock-specific drill bit design chart. The parameters of the drill bit are personalized to the particular rock being drilled. This work allows for determining, in advance, when the bit will require replacement to optimize scheduling of the drilling job in geothermal or fossil fuel wells.
Higgs gives an example of applying the program where various parameters (e.g., the depth of the well, the rotational speed of the bit, the bit fail criteria, bit replacement time) are input to design the drill that will complete the particular job in the least amount of time. In that example, the bit was determined to need outer and inner cutters at different angles alpha and beta, respectively. The depth at which the bit would need to be replaced was also predicted.
This is an example of continuing to explore mixing digital with physical worlds to develop a winning bit design for a particular lithology and rock properties. This is an example of Industry 4.0 where the drill bit is personalized to the specific rock type. While promising, the idea of 3D printing drill bits is still at the research stage and not yet in the field. Industry 4.0 is all about combining digital (data), biological (human) and machine systems to get personalized solutions.
Applying AM to patient-specific orthopedic prosthesis
Higgs provides another Industry 4.0 example involving an AM research project where, instead of a drill bit, an artificial hip joint was personalized to the patient through modeling and 3D printing. Artificial joints often fail due to wear. This particular simulation-based engineering approach involves solving the bioengineering problems of a walking person whose hip joint is modeled with fluid mechanics, solid mechanics, particle dynamics and wear.
4
To highlight the power of simulation-based engineering to test different design scenarios, surface texturing was added to an artificial hip design, and the evolution of wear on the hip joint was simulated as a person walks up and down stairs at different speeds. Research results also demonstrated how engineers could leverage simulation-based engineering to develop patient-specific, osseointegration-friendly implants that promote bone in-growth.
Higgs’ vision is that surgical hospitals will ultimately be able to 3D print just-in-time orthopedic joints that are customized to a specific patient.
Conclusions
By using tribology-aware, AI-based, digital twins of engineering systems,
5 3D printing systems can be integrated into real-world applications. Higgs’ lab has followed these processes in real-world applications for clean geothermal energy and for orthopedic hip replacements using physics-based modeling of tribology, digital modeling of both geothermal and hip processes and experimental validation.
While Industry 4.0 AM is not yet fully realized, Higgs predicts it will change how education and leadership advance going forward. The process will move from the development of a product simulation to the manufacturing of the minimal viable product that is quickly tested and deployed into the marketplace. Higgs believes that our world is moving from mass production to mass customization with everything becoming more personalized through these Industry 4.0 technologies and advanced uses of data. Higgs believes a new generation of tribologists and engineers educated in Industry 4.0 engineering management techniques will be needed to fully realize this brave new world.
REFERENCES
1.
Grant, L.O., Alameen, M.B., Carazzone, J.R., Higgs III, C.F. and Cordero, Z.C. (2018), “Mitigating distortion during sintering of binder jet printed ceramics.” In 2018 International Solid Freeform Fabrication Symposium. University of Texas at Austin.
2.
Carazzone, J.R., Martin, C.L. and Cordero, Z.C. (2020), “Crack initiation, propagation, and arrest in sintering powder aggregates,”
Journal of the American Ceramic Society, 103 (9), pp. 4754-4773.
3.
Wagner, J.J., and Higgs III, C.F. (2021), “Computation of hydrodynamic and capillary phenomena in binder jet three-dimensional printing,”
Journal of Tribology, 143 (5), 051113.
4.
Srivastava, G., Christian, N. and Higgs III, C.F. (2021), “A predictive framework of the tribological impact of physical activities on metal-on-plastic hip implants,”
Biotribology, 25, 100156.
5.
Desai, P.S., Granja, V. and Higgs III, C.F. (2021), “Lifetime prediction using a tribology-aware, deep learning-based digital twin of ball bearing-like tribosystems in oil and gas,”
Processes, 9 (6), 922.
Andrea R. Aikin is a freelance science writer and editor based in the Denver area. You can contact her at pivoaiki@sprynet.com.