Digitalization strategies extend wind turbine life
Jane Marie Andrew, Contributing Editor | TLT Webinars October 2019
Assets are managed more effectively with a two-sided digital platform similar to those used by Uber and Airbnb.
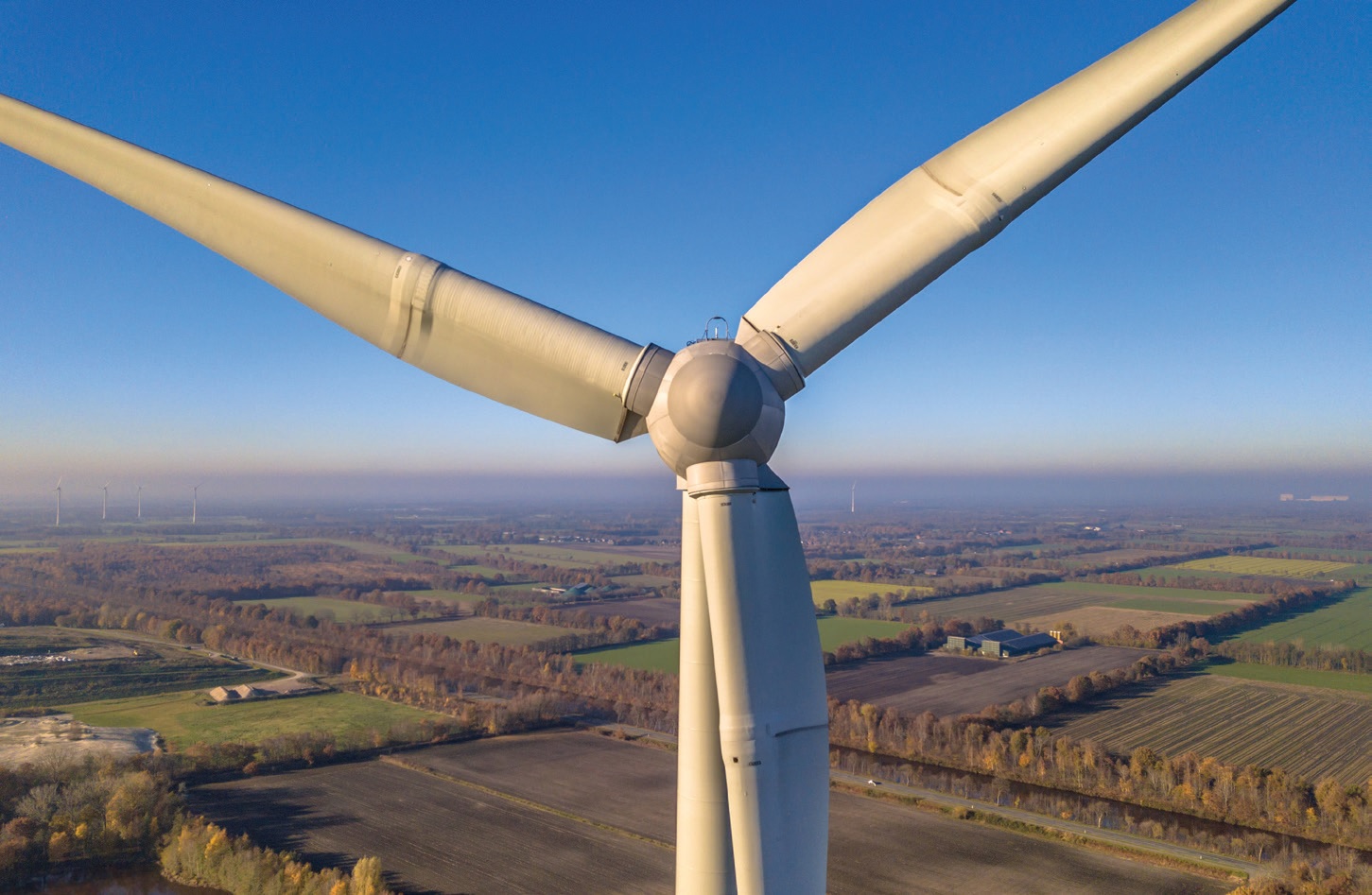
© Can Stock Photo / CreativeNature
KEY CONCEPTS
•
Two-sided digital platforms connect wind energy operators to a broader range of suppliers to aggregate data.
•
Such a platform combines models and field data contributed by both operators and suppliers and uses them to predict failure of specific turbine components.
•
By combining supplier pricing with data-driven failure prediction, the platform can attach a life-cycle cost to individual maintenance actions.
MEET THE PRESENTER
This article is based on a Webinar originally presented by STLE Education on June 26, 2019.
Digitalization of Wind Turbines is available at
www.stle.org: $39 to STLE members, $59 for all others.
Dr. Adam Rasheed is the chief technology officer at Sentient Science where he is responsible for the product strategy, vision and implementation of the DigitalClone platform for the renewables, aerospace and rail industries. He has more than 20 years of experience in early to mid-stage technology development in both industrial and digital domains, including work at GE Global Research.
Rasheed holds a doctorate in aeronautics from Caltech with additional studies in business, economics and management. His technical background spans both engineering and analytics across multiple disciplines, including condition-based maintenance software and analytics, laboratory research in advanced combustion and propulsion and advanced analytics for the aviation and oil and gas industries. He was recognized by MIT’s Technology Review Magazine as one of the World’s Top 35 Innovators and received the Sperry Award from the American Institute of Aerospace and Astronautics for career contributions in aeronautics.
You can reach Rasheed at
arasheed@sentientscience.com.
Dr. Adam Rasheed
The wind energy industry has reached a crossroads in operation and maintenance due to a confluence of factors. The first generation of large-scale turbine installations is reaching end of life, especially in Europe. In addition, end of life came sooner than expected in many cases, as turbines failed at disturbingly high rates. Components with an expected design life of 20 years have failed after eight or 10 years. In the early 2010s, failure rates for gearboxes were especially severe. Finally, government tax incentives are running out.
In general, this situation calls the economics of the whole industry into question. More immediately, it leaves operators struggling to develop realistic and cost-effective maintenance plans to get their assets back to a 20- or 30-year expected life. To ensure that wind energy remains competitive with other energy sources, operators need a broader marketplace for maintenance choices and better tools for understanding the financial implications of those choices.
Anticipating failure
The early failures represent an extreme case of a harsh reality: New designs for industrial equipment are never perfect. Engineering design principles and prototype testing can never cover the full range of operational conditions. The true test comes when the asset is actually fielded. Usually failure rates and failure modes turn out to be different than expected. Suppliers in many industries have worked to bridge this gap by developing condition monitoring systems (CMSs). These systems rely heavily on sensors and data analytics to predict when equipment will fail.
In the wind industry, such systems are usually very good at predicting failures weeks to months ahead of time. That’s good enough for planning and logistics; it helps the operator position equipment at the right time to repair the asset.
But for lowering operating costs, the news is really too late. Sensors can detect cracks only once they reach the surface, but the time to act is when cracks are new—and invisible. Any proactive remediation must start long before a CMS begins to signal trouble (
see Figure 1).
Figure 1. Damage progression cycle. Green: The turbine’s condition remains as installed. Yellow: Cracks have started in the interior of the component. Orange: Cracks reach the surface and begin to affect performance; sensors begin to detect failure. Red: Cracks are severe, requiring immediate decisions about replacement. Dotted green line: Early proactive maintenance during the yellow phase may significantly extend the component’s lifetime. (Figure courtesy of Sentient Science.)
Therein lies the true headache for wind energy operators: They have no consistent, practical, easy process to evaluate the life-cycle costs of different maintenance strategies. Given the complex interactions among depreciation, inflation, lifetime generating capacity and a host of other factors, it is challenging to determine the profitability of a particular maintenance investment made at a particular time—its net present value, in finance terms. Furthermore, when an OEM or a new supplier proposes a new repair strategy, the only way to validate that strategy is to try it out—then wait two or three years. That’s costly.
A solution may be emerging, one that takes its cue from the digital strategy behind the spectacular revolutions that have created the sharing economy.
Digital platforms
In the last decade, long-established industries have been turned inside-out by a type of digital environment called a two-sided platform. Although the term may be unfamiliar, any user of Uber or Airbnb has participated in a two-sided platform. The disruptive power of this type of digital environment may be the force that can bring new solutions for asset management in the wind industry.
The term platform is ubiquitous in IT, but here it has the specific sense used by Geoffrey Parker and the authors of Platform Revolution (
1)—a two-sided platform is a digital marketplace that allows supply and demand to negotiate seamlessly. It is an ecosystem where two sets of participants—consumers and producers—exchange something of value as quickly as possible with as little friction as possible. Within Uber, a driver and rider exchange the value of a ride. Within Airbnb, a host and guest exchange the value of a night’s accommodation. Without question, platforms can lower costs throughout an industry, ideally while also benefitting both sides.
In the wind energy industry, the consumers are turbine fleet operators; the producers are OEMs and subcomponent suppliers. They expect different benefits and need to do different things, but their interests are linked through the physical turbine fleet. That link is key to the all-important first step in building a platform—defining the unit of value being exchanged.
In the approach taken by Sentient Science, the unit of value is a “life extension action.” Such an action is defined in three parts: a description of the action, a market-determined price and a computationally derived life prediction associated with executing the action. The prediction states that if the action is taken, then a specific turbine, operating under specific conditions, will have its life extended by a specific, calculated amount of time.
Once that value is clear, the operator can make an economic life-cycle decision, for example, between a $5,000 option that adds three years and a $2,000 option that adds one year. When the platform allows this kind of apples-to-apples comparison, the operator can say, “Yes, this action that costs me $5,000 today is worth it if I get a three-year life extension.”
Defining the unit of exchange is the central decision in creating a platform, but the success of the platform depends on how seamless it is—that is, how well it eliminates the barriers to making the exchange. When the barriers are low enough, many new producers can enter the exchange, driving down costs and improving efficiency. The Uber platform permitted anyone with a car to become a provider of the unit of value—a ride.
Thus, a true two-sided platform does much more than drive internal efficiency. Rather, it changes cost structures throughout an industry. For the wind industry, it also could mean better access to the best techniques and knowledge to support life-extension strategies.
Related digital trends
The move to industry-wide platforms is part of a larger trend often called Industry 4.0 in Europe (
2) and the Industrial Internet of Things (IIoT) in the U.S (3). It focuses on digitalization and connectivity through the entire industrial process, from design engineering to the factory floor to interactions between factories. The hope is that with these interconnections, participants will be alerted sooner about issues and will be able to respond more efficiently.
A related digital evolution is the move toward digital threads. A digital thread unifies digital records about an asset across functions that traditionally have been siloed, from the earliest steps in product definition all the way through asset management. The aerospace and nuclear industries have used this approach from the beginning, for safety reasons; with advances in computing it is becoming practical for more sectors.
It is important to note how Industry 4.0 and digital thread differ from a two-sided platform. The former strategies have focused mostly on creating efficiencies only within a company and its own vertical supply chain. A two-sided platform, in contrast, broadens the focus—and the potential benefits—to the entire industry.
Platform power
The digital thread concept has two gaps that prevent industry from capitalizing on valuable existing data. In both cases, the gap is between two completely separate organizations. Thus, by definition, both gaps are perfectly suited to a two-sided platform.
The first gap concerns information flow from operator to supplier and the relation of asset management to product development. Once an asset is fielded, the information learned during operation should be used to inform the next iteration of the product. However, the barrier to sharing the operator’s asset information with the supplier is very large.
A two-sided platform supports the interests of both participants here by turning a digital thread into a digital loop. With access to operational data, suppliers spend less to design products that serve their customers better; operators then have a selection of better products. Of course, this kind of exchange does happen today—before Uber, people used taxis—but the process is very inefficient.
The second gap goes in the opposite direction, from supplier to operator. A new product embodies many years’ worth of engineering knowledge. In general, though, that knowledge is closely held within the company or the supplier community. Suppose instead that such models could be made available to operators—in simplified form and with appropriate protection of intellectual property protected. That way, operators would know the intent of engineering design when seeking to understand the failure mechanisms and modes in their fielded assets; by coupling this original intent with data from the field, operators would be able to make better life predictions.
This gap is much harder to close than addressing the design feedback loop. Still, if implemented correctly, so that the relevant information is shared with the relevant people at the right time, all participants can benefit. Data sharing between operators and suppliers allows for more transparency on the expected life of a supplied component, development of better products as suppliers incorporate operational feedback and higher margins for suppliers to reflect the value of extended asset life.
Model support
Everything discussed to this point relates to the outward-facing aspects of a platform: identifying the participants, what is of value to them, what data need to be exchanged. However, the key to actually delivering value lies behind the scenes: building the right models of components and their interrelations. Sentient Science advocates hybrid models. Such models account for not only the engineering and human knowledge used to design the asset but also a range of mathematical, machine learning and data science techniques based on data from the fielded asset.
A key aspect of the modeling approach taken by Sentient Science is creation of a materials science-based digital twin or clone of an entire turbine. This modeling process incorporates science at every level, from the local wind climate to fluid dynamics at contact surfaces (
see Materials Modeling for Predicting Turbine Lifetime).
Remaining useful life
The ultimate goal of all this modeling is to produce a number for the remaining useful life (RUL) of a turbine, component or subcomponent. Said another way, the operator needs to know the date range for when the asset will fail. Recall that part of the value added by the two-sided model is quantification of the life extension that can be expected from a specific action on a specific turbine under specific conditions.
Sentient Science is developing methods to arrive at this number using a hybrid approach to combine material science with data science and recent field observations within a damage progression framework (
see Figure 2). This framework is designed to provide a view that looks at condition years ahead (
see Damage Progression Framework for Quantifying the Date Range of Expected Failure).
Figure 2. Hybrid models merge basic science, brute force number-crunching and (not least) human observation.
© Can Stock Photo / kentoh • cgteam • piedmont_photo
Damage progression framework for quantifying the date range of expected failure
To quantify the expected life extension of a component or subcomponent within a hybrid modeling approach, Sentient Science is exploring damage progression frameworks, presented as graphs of increasing damage over time (
see Figure 3). Figure 4 shows the extensive universe of data and models needed to generate such damage progression curves. This type of data presentation is used to compare predictions of remaining useful life from various models and various assumptions.
Note that even a statement about the current health of the asset is a prediction. It is based on the model of damage propagation plus the last known observations, such as a visual inspection or a vibration inspection. This health state could be verified through an inspection.
A second important note is that each field observation has an uncertainty (because it is performed by a human) and so does each model. Thus, this whole analysis becomes a framework for quantifying uncertainty.
With appropriate assumptions about what operating conditions will be like in the future, the model is projected forward to determine the date at which the predicted damage crosses the failure threshold. The uncertainty distribution associated with this date gives 10th and 90th percentile values for remaining useful life.
As the component approaches end of life, the uncertainty spread will narrow. At this point, condition monitoring systems are suitable. The true value of a damage progression framework is to provide a defensible estimate years in advance, despite uncertainties.
Figure 3. A damage progression framework yields a date range within which failure is expected (remaining useful life probability distribution function [RUL PDF], dotted curve at upper right). The y-axis is some measure of damage (for example, the length of a crack), and some threshold value of y is defined as failure. The solid vertical line is today’s date for the particular asset. (Figure courtesy of Bolander, N., Qiu, H., Eklund, N., Hindle, E. and Rosenfeld, T. (2009), “Physics-based remaining useful life prediction for aircraft engine bearing prognosis,” Annual Conference of the Prognostics and Health Management Society, paper 110. Available at www.phmsociety.org/node/110.)
Figure 4. Data relationships for estimating current asset state and predicting the remaining useful life. (Figure courtesy of Sentient Science.)
Materials modeling for predicting turbine lifetime
The following steps outline how Sentient Science arrives at materials science-based predictions of the lifetime of an entire turbine (
see Figure 5).
1.
An aeroelastic model accounts for how local wind conditions produce turbine motion. Turbine motion is then coupled to the control system model, which manages both the amount of electricity generated and the loads on the tower and its components. By combining the mechanics, conditions and control system behavior, the model can generate aeroelastic load data.
2.
These loads are fed into a dynamic simulation of the drivetrain.
3.
Results from the overall drivetrain model are used to calculate local time-resolved loads on the bearings and the gears.
4.
Next, simulation moves another level deeper to microgeometry models of the bearing or gear itself. For a bearing, the model would include the profile of the inner race, the number of balls and the material properties. The simulation yields time-resolved local loads at the critical point defined for this system. In a bearing, the critical point would likely be the contact between one ball and the inner race.
5.
Going still deeper, the next step is laboratory materials testing on an actual component. For a bearing, this work includes mechanical testing to determine its fundamental properties and microscopy to assess essential microstructure features and composition. These experimental results form the basis of a material model—a computational representation of the material structure and properties.
6.
The material model informs a contact model of electrohydrodynamic lubrication (EHL) to clarify the loads in detail.
7.
All of the results are processed through a component life prediction model, which runs on a high-performance computing cluster.
All these steps are done for every component on the bill of materials from a turbine manufacturer. Finally, Monte Carlo simulations for various material property and loading distributions yield Weibel failure rate curves, such as those shown in Figure 6.
Figure 5. A digital clone or digital twin model captures science and engineering knowledge at every scale of the turbine, from the local wind climate to fluid dynamics at contact surfaces. (Figure courtesy of Sentient Science.)
Figure 6. Detailed, multi-scale material modeling yields Weibel failure curves to support long-range asset management. (Figure courtesy of Sentient Science.)
Predictions in practice
The overall goal of the platform is to give users easy access to information that helps them do their work. The following is an example of how an asset manager might drill down to a specific action.
Everyday monitoring starts with a watch list covering the entire turbine fleet; the list ranks the turbines by failure risk. It highlights units expected to fail within the next few months and gives the likely date range. The manager can click on an at-risk asset to locate the subcomponent that is probably responsible for the risk ranking.
Now that the manager knows which turbine to worry about and which drivetrain component needs attention, the next decision is choosing the maintenance action with the best life-cycle cost. The manager can access a list of maintenance actions for the component. This list is populated with offers by various suppliers to the platform and includes an estimate of lifetime extension for each action. When the manager chooses a set of actions, the effect on life-cycle cost is calculated and displayed visually. All these interfaces are enabled by the kind of deep-level engineering modeling previously described.
Summary
Taking a cue from the retail and service sectors, industry and manufacturing are embracing two-sided platforms that connect large pools of consumers and producers. Given the history of platforms such as Amazon, Airbnb and Uber, major shifts in the manufacturing landscape could follow.
In the wind energy industry, a two-sided platform holds promise for improving turbine lifetime by connecting turbine fleet operators with a broader pool of suppliers and maintenance options. This alone has the potential to improve efficiency and reduce costs throughout the supply chain.
Beyond these economic advantages, a platform could advance the industry overall by removing technical and intellectual property barriers that prevent operators and suppliers from sharing data. With access to large quantities of field performance data, suppliers can design better components. With access to scientific and engineering models of component materials and performance, operators gain a better understanding of failure modes.
REFERENCES
1.
Parker, G.G., Van Alstyne, M. and Choudary, S.P. (2016),
Platform Revolution: How Networked Markets Are Transforming the Economy—And How to Make Them Work for You, New York, N.Y.: W. W. Norton & Co.
2.
Baur, C. and Wee, D. (2015), “Manufacturing’s Next Act,” McKinsey & Co. Available
here.
3.
The “4.0” of the name is a reference to the three preceding phases of industrialization: large-scale machinery, mass-production and automation.
Jane Marie Andrew is a freelance science writer and editor based in the Chicago area. You can contact her at jane@janemarieandrew.com.