Holes in the data
Evan Zabawski | TLT From the Editor May 2019
The extant information doesn’t always tell the whole story.
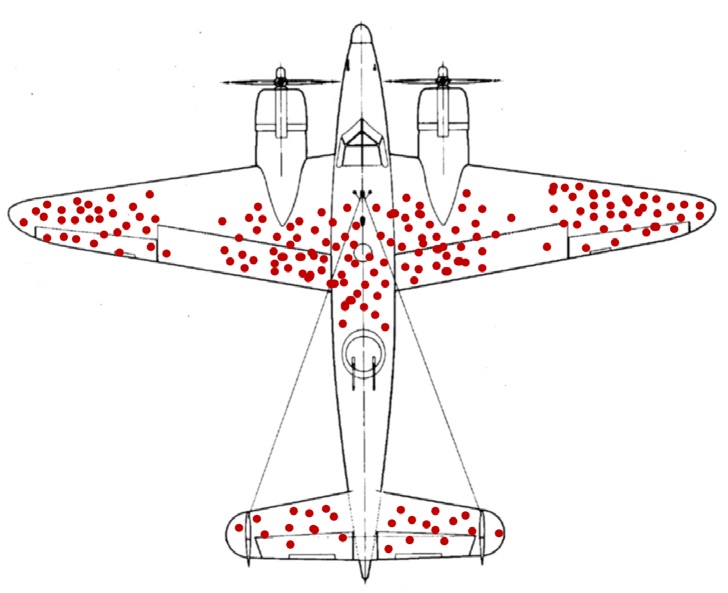
To see how easy this error is to commit, look at the diagram and choose four locations to add armor.
Photo courtesy of Wikimedia Commons. Author: McGeddon.
A common error during data interpretation is forming a conclusion without understanding if the data has been filtered by a form of natural selection. In some cases, the absence of supporting data may be more telling, but the difficulty lies in knowing if data has been filtered out or if there is simply no supporting data.
A classic literary example of no supporting data can be found in the Sherlock Holmes story The Adventure of Silver Blaze. In the story Holmes must locate the eponymous race horse, which has gone missing from its stable and solve the mystery of how its trainer was killed in the process.
In an exchange with Scotland Yard detective Gregory, Holmes is asked, “Is there any other point to which you would wish to draw my attention?” to which he replies, “To the curious incident of the dog in the night time.” Gregory comments, “The dog did nothing in the night-time,” which Holmes acknowledges with, “That was the curious incident.” The plot hinges on the fact that the dog did not bark, suggesting the person who stole the horse was known by the dog, and thus Holmes concludes the theft to be an inside job by the horse’s trainer (who was killed by the horse).
A great example of missing data is a paper titled A Method of Estimating Plane Vulnerability Based on Damage of Survivors published by the Center of Naval Analyses. The author, statistician Abraham Wald, was a member of The Statistical Research Group based at Columbia University during the Second World War and supported by the Applied Mathematics Panel that was formed to solve mathematical problems related to the war.
The paper discussed the error of basing a decision on future armor plating of aircraft from damage data gathered from returning aircraft. To see how easy this error is to commit, look at the diagram and choose four locations to add armor. Did you pick both wingtips, the tail and the fuselage over the wings? Wald chose the cockpit, both engines and the fuselage between the wings.
Wald’s decision was based on the point that the damaged areas in the diagram only represented all the aircraft that survived their missions, and he argued that the undamaged areas represented sections that must have resulted in plane crashes when they received any damage. This has become known as survivorship bias.
The same phenomenon occurs in in-service fluid analysis, particularly in samples submitted post-failure with a desire to identify a root cause. Given that 95% of the wear debris that could provide useful insight into machinery condition is caught in the filter and never ends up in the fluid sample, data from the analysis may be subject to survivorship bias.
Example: if a sample from a rotating machine equipped with journal bearings and filtration shows no sign of Babbitt wear, is the inference that the bearings are in good order correct? The question that should be asked is does the sample not indicate wear because there was no wear, or because the wear on the journal bearings was so severe that the wear debris was trapped in the filter and the fluid sample simply did not contain the wear particles?
It is frequently suggested that one should never pull fluid samples downstream from a filter due to the possibility of some loss of data, but the reality is that a sample upstream from the filter is not significantly different in a constantly circulating system—the sample is only missing as much wear as would be generated in the time it takes the fluid to circulate once through the system.
A better idea would be to encourage routine inspection of the internal media of filters for evidence of wear particulate any time a filter is replaced. When signs of wear debris are detected, the filter should be remitted to a lab for filter debris analysis to determine the size and shape, and consequently the severity, of the wear debris. This minimizes occurrences of failures preceded by normal-looking reports.
Evan Zabawski, CLS, is the senior technical advisor for TestOil in Calgary, Alberta, Canada. You can reach him at ezabawski@testoil.com.