Complexity in ISFA (in-service fluid analysis): Part XXVII
Jack Poley | TLT On Condition Monitoring May 2016
Mining for dollars Part III—making it easier on the intelligent agent.
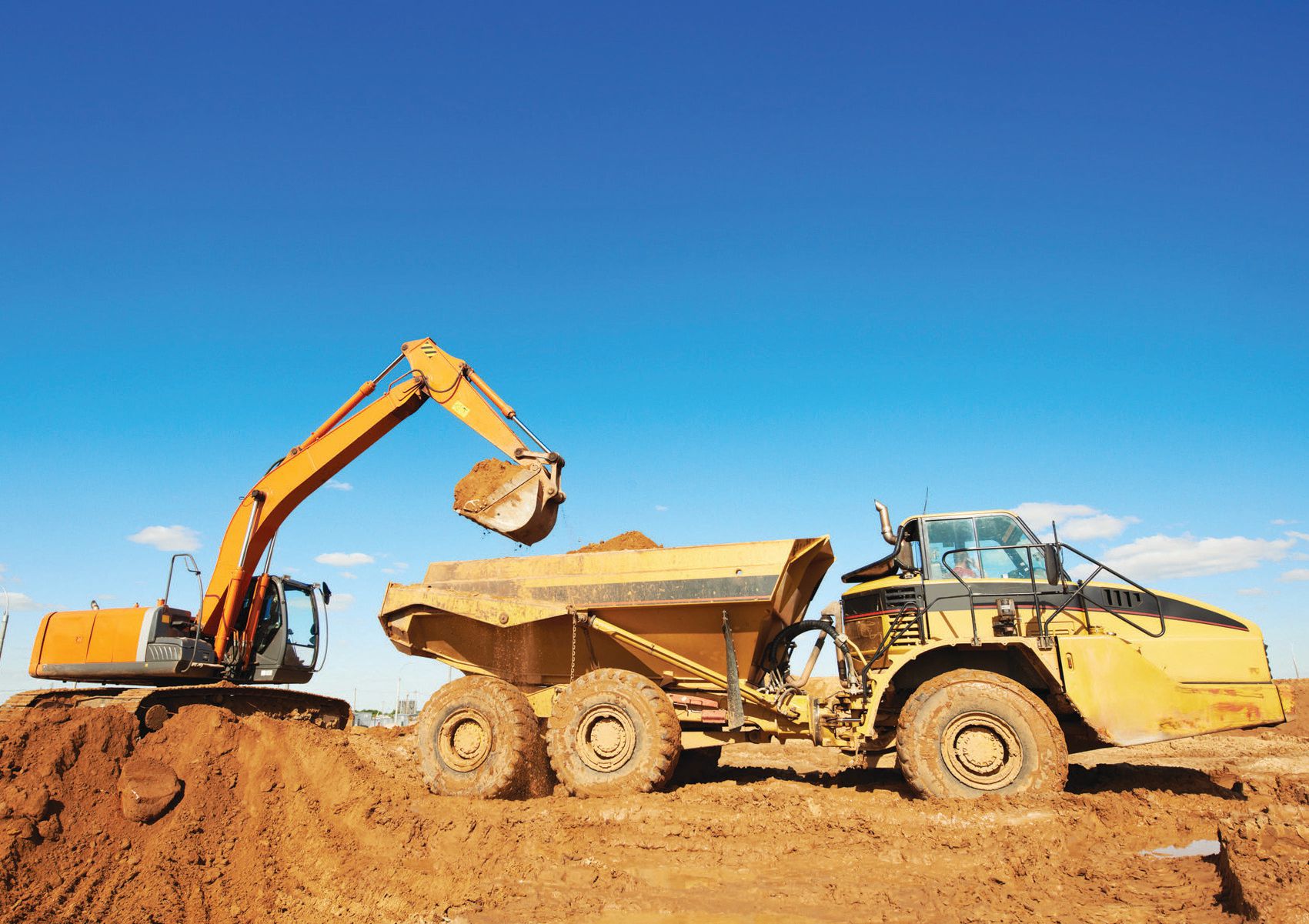
© Can Stock Photo Inc. / kadmy
IN THE LAST TWO COLUMNS, I RESPECTIVELY DISCUSSED the overview of ISFA information that should be in your fluid analysis database. Then I elaborated more on the component type (Comp-Type), that is the cornerstone of the sump’s description (i.e.,
what is it?). We pick up from there.
•
Component MFR/model.* Please, no
model should be provided without an MFR to qualify it—such instances will be ignored by a properly programmed ISFA.
•
Application. I came close to stating the application may be more important in the evaluation hierarchy than model, maybe even component MFR. However, it’s only when the application is truly exceptional that this becomes the reality.
For example, suppose an MFR (“X”) makes an engine model that is quite versatile and can be used in buses, over-the-road tractors, mining equipment and generators. Surely one can quickly recognize that wear metal levels and generation rates, along with other important fluid test data, are not going to be the same. It would be, then, a lapse in judgment to have only a solitary table of boundaries (TOB) for various test data. The application should surely be differentiated, reflecting anticipation of substantial differences in test results for the same datum type, particularly when the least stressful application is in play versus the most traumatic.
Everyone gets it for off-highway: dirt. One must allow for inherently higher levels of Si in the lube (maybe Al as well) and evaluate accordingly. We’re not going to get into detail as to whether or not a naturally dusty environment can be thwarted with best diligence; it can. But I assure you, Si is generally higher for most operations of that ilk. Fe, Al, Cu and Pb follow. The effort necessary to lower Si to on-highway (lower) levels simply isn’t made. One can probably argue it is too costly to achieve. Sometimes true, other times perhaps not.
What about the application where the component is coddled? How about the gen set? Constant RPM, comfortable load, roof over its head. So we say, yes, the wear levels and rates should be lowest of all, compared to most applications. So is there nothing to worry about? What if a wear problem does occur (it can still happen, of course), but if one is still looking at smaller numbers than this component presents in other middling applications, with respect to stress, might we not look at these data in a more specialized manner? How about trending beneath the limits set in the TOB so that significant changes can be noted in spite of lack of limit flagging? It can’t hurt—I’ve seen that technique save a few components over the years. Intelligent agents (IAs) can do that sort of thing as a routine, trivial exercise. The human simply has to inform the IA as to what advice it might render.
This latter example is very analogous to the medical environment for humans; suppose one has a cholesterol value of 220 (usually considered abnormal) and it goes to 230 over an interval. Suppose as well another person has a cholesterol level of 120 and it goes to 180. Who’s possibly in greater short-term trouble?
Change matters. Every evaluator of any competence will always agree, but some are mired in a mantra-like belief that trending is better than limits. Not necessarily true—best to do it all. We’ll talk about trending in more detail soon.
•
Wild card (if the ISFA has this category). Yet further differentiation when useful, e.g., perhaps a new alloy is being tested for ring sets in a diesel engine, but the generic model terminology is still being used. This designation allows easy separation for comparison purposes.
The following are recommended for
decoupled consideration from the above.
•
Lube MFR/brand.* Please, no
brand should be provided without an MFR to qualify it—such instances will be ignored by a properly programmed ISFA.
•
Grade. Keeping this property as a separate field greatly minimizes the number of times that a brand has to be entered. Less to maintain, less chance for error.
•
Filter MFR/brand.* Personnel making entries in databases routinely make typos and designate incorrect categorizations of models (component MFRs) and brands (lubes and filters), such that the same item is misrepresented no less than a dozen times or more. As well, many submitters of sample information and samples make those same errors, in effect, training the lab personnel to be unwittingly careless. Even if one is using dropdown menus to stifle yet more additions of an incorrect nature, the proliferation is nothing short of amazing—and ruinous. There is no practical way to resolve this without writing some code to go in and make changes, or aliasing all deemed (and you will miss some while wrongly inferring others to be worthy of aliasing) to accept alternate variations.
I mentioned several columns before that this kind of sloppiness doesn’t often result in a penalty if one is evaluating manually because humans can override such errors/noise intuitively—but this is about the last place left for humans to excel over computing, in the routine processing area of fluid test data evaluation. And in this hypothetical discussion, bypassing the actual model and therefore defaulting the differentiation of models altogether, it dilutes the depth of understanding that might be gleaned when a given model behaves quite differently versus one with a similar model designation. The human will never know, and the IA will never know either, unless the human instructs it more consistently.
And this is the last time I’ll apologize for the original database setups in most current ISFA participants. It wasn’t necessary—or even useful—nor could one know, unless one had a Ouija board in days past, but quality database preparation is now essential if one wants to achieve the best results possible from ISFA.
Fact: Humans have run their course as
routine evaluators—IAs are far superior to evaluating routine sample test data results that suggest little or no trauma is indicated. Unlike humans the IA is relentlessly consistent and virtually error-free or quickly correctable when an error is identified. Boring, but most effective. The human’s value, essentially a
promotion, is in informing the IA to the best of her/his experiences and abilities—together with reviewing and vetting (primarily) the critical reports in order to build accuracy with consistency—and ultimately being able to provide confidence levels when a comment is rendered. This is where things have to go. Heuristic knowledge is the backbone of domain expertise; domain expertise is what informs IAs to the best advantage. This is what maximizes production and generates savings. Yes, it’s for sure about money.
What’s missing? Glad you asked:
Feedback—accurate reporting of findings, whether recommended or not, along with actions taken—again—whether recommended or not. This is the only way an IA can learn. Such information in the hands of a domain expert is akin to gold. Solid vetting can take place and commentary can be bolstered to achieve the maintenance organization’s trust.
Over the next article or two we’ll summarize the last three columns and try to throw feedback into the consideration hopper and see what a world-class ISFA program can look like. I also will elaborate on the decoupling of lubricant/ filter evaluation (initially) and connecting it in the overall evaluation.
*These areas (lubricants/fluids, the most) are the Achilles heel of database management due to the exponential growth of component models and lube MFR brands. Filter brands less so but usually ignored altogether. Yet many people have come to me wanting to compare MFRs, models, lubes and brands and even filters. Go figure.
Jack Poley is managing partner of Condition Monitoring International (CMI), Miami, consultants in fluid analysis. You can reach him at jpoley@conditionmonitoringintl.com.
For more information about CMI, visit www.conditionmonitoringintl.com.