Complexity in oil analysis information
Jack Poley | TLT On Condition Monitoring November 2011
A detailed database ensures you’re ready to evaluate with good expectations.
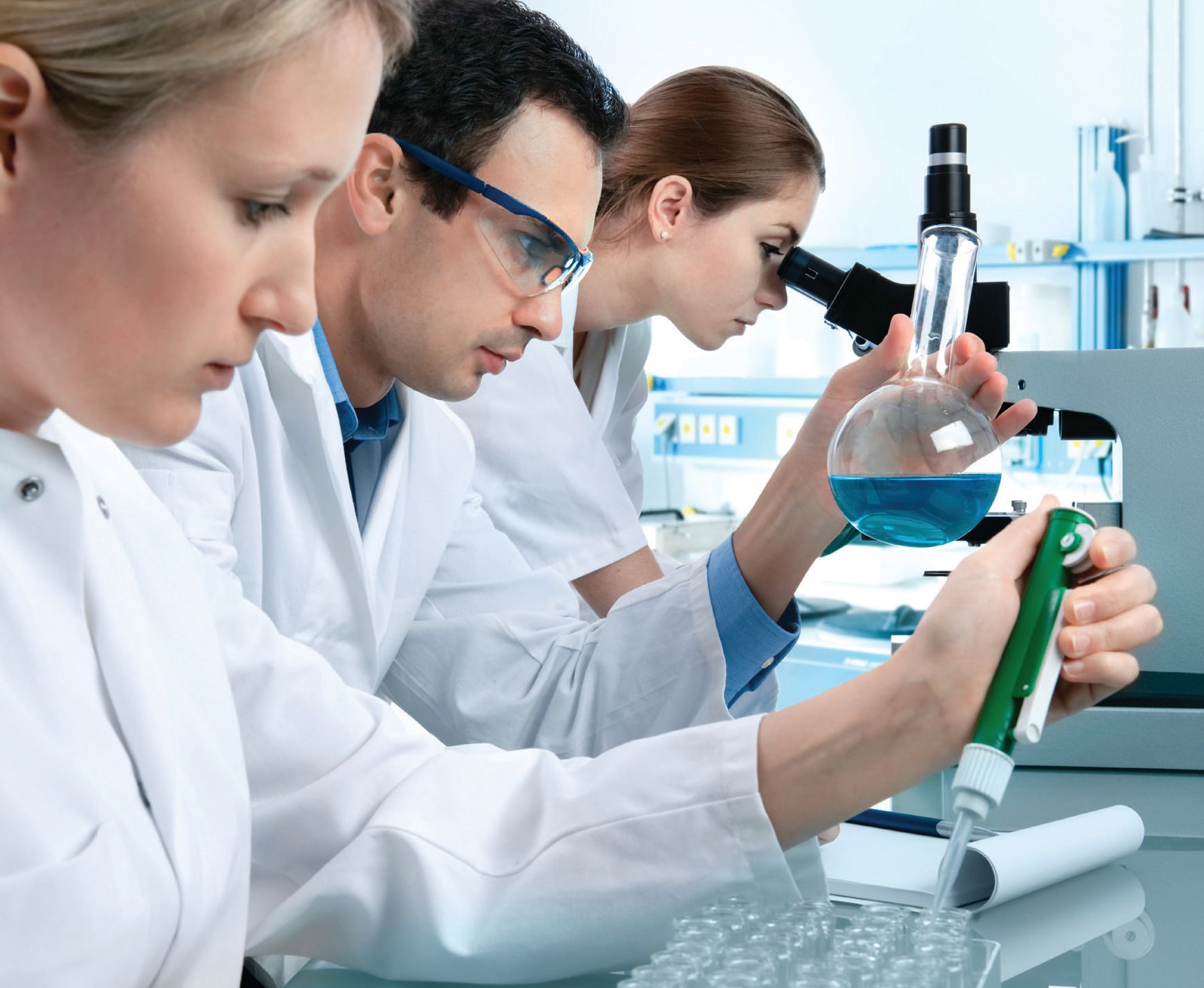
www.canstockphoto.com
As previously noted, 21st Century Oil Analysis (OA) is upon us, and it is a seminal point. It’s not that this century is occurring that is particularly important, it’s that the beginning of this century coincidentally marks the onset and developing efficacy of a three-tiered system for modern oil analysis that, in turn, provides new test methods, integration and challenges in one’s efforts to evaluate test data.
When OA shifted from lubricant contamination and degradation inspections to the inclusion of wear metals analysis, the dynamic changed geometrically, for example:
•
Primary Big 4 wear metals (Fe, Al, Cu, Pb), Si and, perhaps, Sn are minimal wear inspection requirements.
•
Add contaminant metals, e.g., Si, B, Na, K, etc.
•
Then additive metals, e.g., Ba, Ca, Mg, P, Zn, etc.
•
Then add ancillary metals for more specific insight into a specific wear point, e.g., Cr, Ni, Mo, Ti, Sb, Co, W, etc.
•
Then note that numbers of the above metals can have two, three, even four chemical configurations concurrently (I call these Chameleon Metals)…and one has the ingredients for a complex undertaking, the
Evaluation.
We’re not nearly through with the considerations. Let’s introduce and confirm some terms for reference. Let’s start at the beginning of the OA cycle, once a decision has been made to participate, assuming (and, sadly, wrong too often) that everyone who participates in an OA program from an influential position is fully aware of the following needs and does something about it, with respect to proper and sufficient equipment/component parameters in his/her database.
•
Equipment. This is the item that houses or hosts the component. It’s the macro-item, e.g., a bulldozer, truck, manufacturing plant, boat. Sometimes the equipment is the component: a stand-alone gas compressor or a windmill. If the component is not identified, the equipment may be proposed as the component until further clarification.
•
Component. This is the item being sampled. The component is where the SPID (Sample Point I.D.) exists. If the equipment is not identified, the component may be proposed as the equipment.
•
Component Type. This is the primary essential piece of information for evaluation, and it’s not optional. What is this machine monitoring? Hydraulics don’t work like gas turbines, nor do they necessarily possess the same parts or metallurgy. The intelligent agent I employ to serve our customers has more than 100 different types and subtypes of components where the mechanical configuration is substantially different and where such consideration is mandatory in order to achieve a credible evaluation when data are abnormal.
If a component type has a subtype that clearly differentiates it from a generic, it must be clarified. An example of how even well-intentioned sample submitters do not maintain a fully descriptive component type is the term hydraulic. A sample is submitted from a hydraulic system. While this is better than nothing, it is certainly well short of being adequately descriptive. What kind of hydraulic (pump or motor) are we observing: gear, vane, axial piston, etc.?
Again, the sample-taker or certainly the report recipients understand what kind it is. But if this information never makes it to the evaluator, be it human or intelligent agent, a multiplicity of comments may have to be spewed out to cover the bases, e.g., “If this is a gear pump,
do this,” “If this is a vane pump,
don’t do that,” and so forth. Humans and software robots alike will not commit to anything specific, and should not, if they’re paying attention.
•
MFR/Model. I once managed a program for a very large truck operator who had literally thousands of truck engines represented (differentials and transmissions were rarely sampled). When their equipment files were first submitted to inform the database and initiate the program, many manufacturers were listed such as Peterbilt, Navistar or Kenworth. Navistar trucks usually have Navistar engines in them, whereas several manufacturers can power Peterbilt and Kenworth trucks. What’s wrong with this picture? We know the equipment (the truck) MFR, but we don’t know the component MFR (Caterpillar, Cummins, Detroit Diesel, Volvo and the like). So we were obliged to treat samples of such limited provenance as generic diesel engines, depriving us of precise wear metals source knowledge.
With thousands of samples of each engine type actually in the database, it was paramount that the engine MFRs be derived, and we wrote code to accomplish this, including sorting out individual models. The wear tables that followed were significantly different in some instances, easily justifying the exercise and allowing for proper data rating and flagging. But it was a lot of work that could have been avoided with diligent database management prior. More on that later.
www.canstockphoto.com
LUBRICANT INFORMATION
•
MFR: Pick an oil company.
•
Brand: This is the marketing name for the MFR’s oil.
•
VIS Grade: This is the expected VIS of a sample.
Ideally each of these three items is provided in a separate column in the database. Why? Because it’s easier to construct rules and protocols for evaluating additive packages and establishing correct baselines for additives and their excursions, including VIS deviation versus new oil test references.
With most files I’ve seen lately that are rife with incomplete and misplaced information, even misspellings, plenty of algorithms had to be written to get the lubricant aspect constructed correctly.
Well, you get the idea about component identification, right? It’s got to be as deep as the component’s difference from another component. Sometimes generic treatment works, but numerous times (and as often as not) it doesn’t. Then there’s the lubricant information details, which tend to get even more neglect than the component type. Good insight is being lost when information is short-changed.
I used to feel that laziness and sloppiness were the two drivers for poorly maintained databases, with respect to OA needs. However, I’ve since come to the conclusion that most of the databases existed before OA was a regular part of maintenance practices. These databases were sufficient for most needs at the time—however inconsistent or incomplete—until OA came along and essentially demanded more and with greater consistency. So it was benign naivety, coupled with no pressing or evident need to be meticulous, that were the real culprits, resulting in databases with numbers of errors and flaws in the categorization of data and the way the data were (inconsistently or erroneously) entered.
So what to do? A good sanitizing exercise can fix most OA databases. This is the recommended action prior to loading data into a lab’s or consultant’s computer to begin (or transfer) a program. One also can have the consultant perform the exercise, when specialized software tools are available to facilitate the task. The point is: take the care to get your information in order so that a quality evaluation can be repeatedly rendered.
It’s the evaluation that is the OA goal.
www.canstockphoto.com
The last need before we’re ready to evaluate is tests selection:
•
Know which tests make the most sense for the component under scrutiny.
•
Make sure those tests are being performed.
Following are the most popular test instruments for OA, largely because of their breadth of coverage for most component types:
1.
UV spectrometer (SOA): wear, contaminant, additive metals
2.
VIS baths (40 C and 100 C, primarily)
3.
Infrared spectrometer (mostly FTIR)
•Soot (diesels)
•Oxidation (marginal when synthetics are in use)
•Nitration (engines, primarily)
•Water (coarse)
•Glycol (coarse or POS/NEG)
•Fuel Dilution (diesels, very coarse)
4.
Particle Counter (hydraulics, primarily, but adaptable).
Unless your components are very singular in nature, it is likely these tests are mandatory in your OA program. But there are certain exceptional component types and applications, for example:
•
Diesels. If this is your main component type or if you simply have a lot of them, you are or should be concerned about fuel dilution. You will do best to demand gas chromatography for accurate fuel dilution measurement.
•
Gearsets (unfiltered). You are not fully spanning the needed size range with respect to particulates, using a UV spectrometer whose sensitivity ends well below 10 µ particles. A coarse metals inspection with instruments such as PQ (Particle Quantifier [Fe]) or fdMplus (Ferrous Debris Monitor, ppm) or DRF (Direct-Reading Ferrography or LaserNetFines) is needed, in conjunction with SOA to check for the onset of fatigue-oriented wear. More on this later.
•
Hydraulics or Refrigeration Compressors. You will need to monitor water more rigorously than with a hot plate sputter or FTIR coarseness. Karl Fischer water (ppm) should be employed for much better sensitivity.
With a cleansed, well-organized database and proper tests, we’re ready to evaluate with good expectations! However, we’ve not discussed the complexity of the evaluation, just the complexity of the minimum information and testing setup.
Jack Poley is managing partner of Condition Monitoring International (CMI), Miami, consultants in fluid analysis. You can reach him at jpoley@conditionmonitoringintl.com.
For more information about CMI, visit www.conditionmonitoringintl.com.